Jürgen Schmidhuber
Tanja Schultz
Walter Senn
Henry Markram
Jürgen Schmidhuber
IDSIA USI-SUPSI, Switzerland, and KAUST AI Initiative, Saudi Arabia
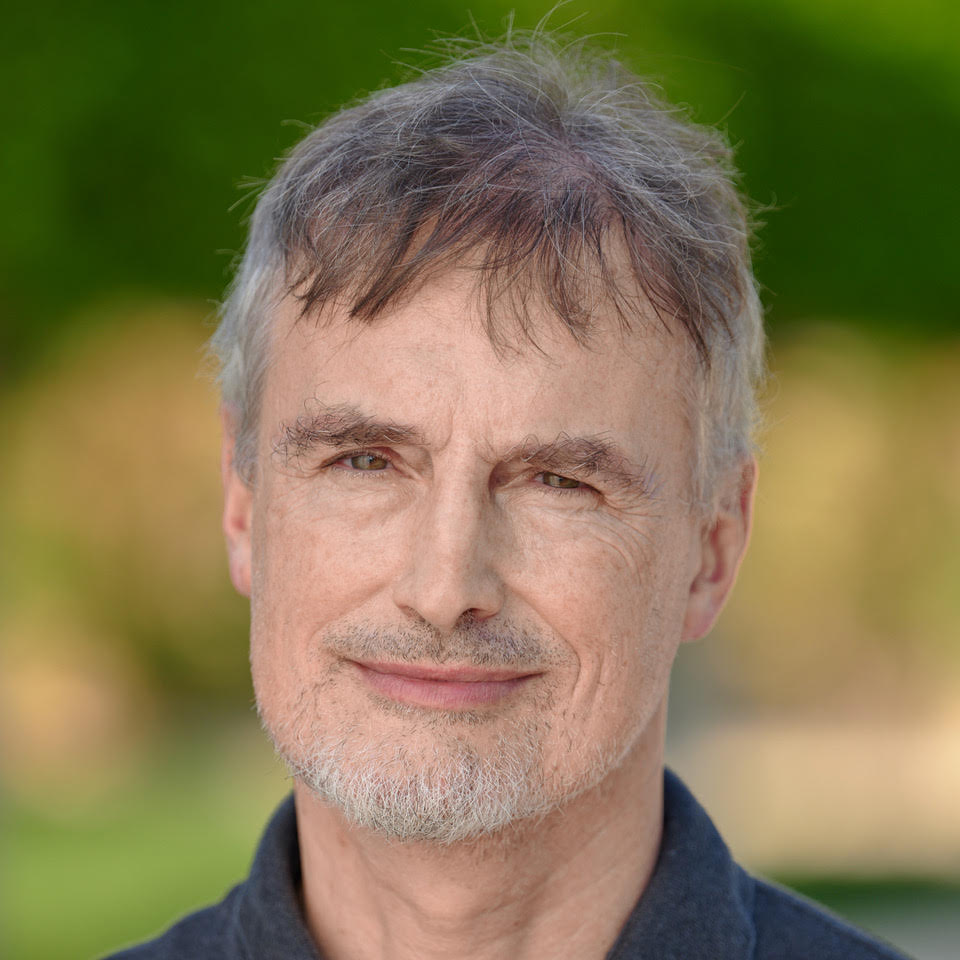
The New York Times headlined: “When A.I. Matures, It May Call Jürgen Schmidhuber ‘Dad’.” Since age 15, his main goal has been to build a self-improving Artificial Intelligence smarter than himself, then retire. His lab’s deep learning artificial neural networks have revolutionised machine learning and A.I. By 2017, they were on over 3 billion smartphones, and used billions of times per day, for Facebook’s automatic translation, Google’s speech recognition, Google Translate, Apple’s Siri & QuickType, Amazon’s Alexa, etc. Generative AI is also based on his work: he introduced artificial curiosity & generative adversarial networks (1990, now widely used), self-supervised pre-training for deep learning (1991, the “P” in “ChatGPT” stands for “pre-trained”), unnormalised linear Transformers (1991, the “T” in “ChatGPT” stands for “Transformer”), and meta-learning machines that learn to learn (since 1987, now widely used). His lab also produced LSTM, the most cited AI of the 20th century, and the LSTM-inspired Highway Net, the first very deep feedforward net with hundreds of layers (ResNet, the most cited AI of the 21st century, is an open-gated Highway Net). In 2006-2010, he published the “formal theory of fun and creativity.” Elon Musk tweeted: “Schmidhuber invented everything.” He is recipient of numerous awards, Director of the AI Initiative at KAUST in KSA, Scientific Director of the Swiss AI Lab IDSIA, and Co-Founder & ex-President of the company NNAISENSE. He is a frequent keynote speaker at major events, and advises various governments on A.I. strategies.
Homepage of Jürgen Schmidhuber
Tanja Schultz
University of Bremen, Germany
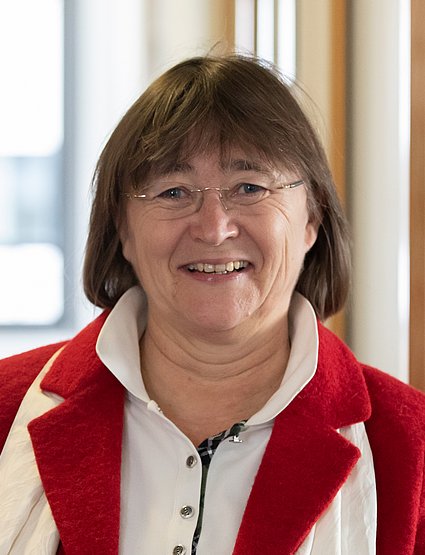
Tanja Schultz is a professor of computer science at the University of Bremen, director of the Cognitive Systems Lab and speaker of the DFG AI Research Unit “Lifespan AI” and the high-profile area “Minds, Media, Machines”. She received her doctoral and diploma degree in Informatics from University of Karlsruhe, Germany in 2000 and 1995 respectively. In 2000 she joined Carnegie Mellon University in Pittsburgh, Pennsylvania where she held a position as Research Professor at the Language Technologies Institute until 2020. From 2007 to 2015 she was a Full Professor at the Department of Informatics of the Karlsruhe Institute of Technology (KIT) in Germany before she joined the University of Bremen in April 2015. Since 2007, Tanja Schultz directs the Cognitive Systems Lab, where her research activities focus on biosignal-adaptive cognitive systems with a particular emphasis on the acquisition, processing and decoding of multimodal biosignals in real-time with the aim of interpreting human behavior and predicting human needs in the context of communication and interaction with cognitive systems and robots. Tanja Schultz received several awards for her work including the Allen Newell Medal for Research Excellence from Carnegie Mellon, the Alcatel-Lucent Research Award for Technical Communication, the Plux Wireless Award, the Otto-Haxel Award, and two Google Research Faculty Awards. She is a fellow of ISCA, EACS, AAAI, and IEEE and has co-authored about 500 articles published in books, journals, and proceedings.
Walter Senn
Institute of Physiology, University of Bern, Switzerland
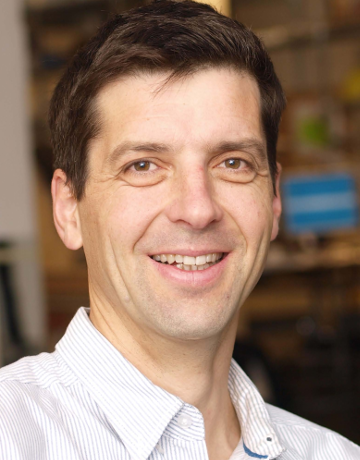
Walter Senn is Professor for Computational Neuroscience and co-director of the Department of Physiology, University of Bern. He studied Mathematics and Physics, with a PhD in differential geometry and calculus of variation (1993). During his PhD he studied dynamical systems at Lomonossov University in Moscow, and he got a degree as a high school teacher from University of Zurich. Before joining the Department of Physiology (1999), he was at the Department of Computer Science at University of Bern, spending postdoc time at the Hebrew University, Jerusalem (I. Segev), and the Center for Neural Sciences, New York University (J. Rinzel). Using mathematical models of synapses, neurons and networks, he investigates how cognitive phenomena such as perception, learning and memory can emerge from neuronal substrates. His recent interests are devoted to translate principles of cortical computation into algorithms for neuromorphic systems (with M. Petrovici), inspired by progress in AI (partly with Y. Bengio).
Henry Markram
Swiss Federal Institute for Technology, Switzerland
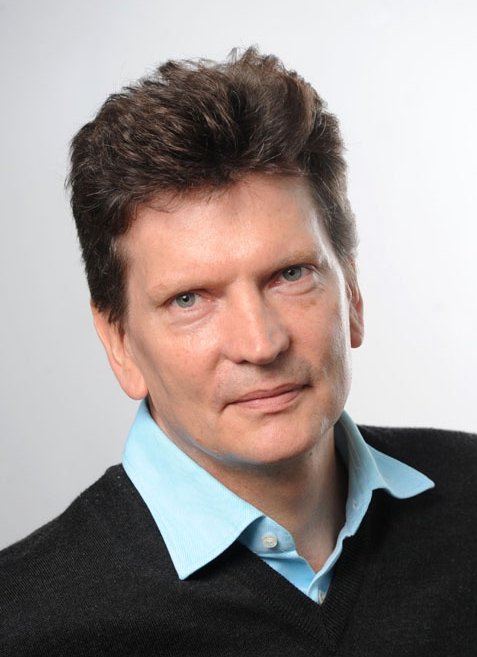
Henry Markram is a professor of neuroscience at the Swiss Federal Institute for Technology (EPFL), director of the Laboratory of Neural Microcircuitry (LNMC) and the founder and Director of the Blue Brain Project. After earning his PhD at the Weizmann Institute of Science, with distinction, he was a Fulbright scholar at the National Institutes of Health, and a Minerva Fellow at the Max-Planck Institute for Medical Research. In 1995, he returned to the Weizmann Institute, becoming an Associate Professor in 2000. In 2002, he became a full professor at EPFL. Markram’s research has focused on synaptic plasticity and the microcircuitry of the neocortex, in which he has discovered fundamental principles governing synaptic plasticity and the structural and functional organization of neural microcircuitry. Other key discoveries include the concept of Liquid Computing and the Intense World Theory of Autism. In 2005, he launched the Blue Brain Project to reconstruct and simulate the mouse brain. Markram is also the founder of the Brain Mind Institute and the founder of the European Human Brain Project, one of two ten-year one billion Euro Flagship Projects selected in January 2013 by the European Commission. Markram has received numerous awards including the Shannon Visionary Award from Bell Labs and the International Hebb Award from the International Neural Network Society.